Artificial Intelligence
Process data has been determined and recorded since the beginning of industrial plant automation. We use artificial intelligence methods, in particular machine and deep learning, to create data-driven models from your operating data. These fulfill a variety of application purposes, support your employees in operation and increase the efficiency of your system in a comprehensible way.
Do you have problems for which conventional solutions are not sufficient? Do you need support in predicting developments or recognizing unusual conditions? We offer solutions from the field of machine learning that can help you with this.
Let’s work together to find a solution!
Would you like to find out more? Should we call you or would you like to receive further information by e-mail?
I am happy to be there for you!
Petra Steinmetz is your expert when it comes to artificial intelligence.
Use case prediction of power consumption
In district heating networks, the energy demand must be covered at all times. In biomass (heating) power plants, the biomass boiler often cannot react quickly enough to fluctuations in demand and peak loads. In order to be able to meet the demand, a fossil fuel-fired peak load boiler is usually used. On the one hand, this is cost-intensive and, on the other, causes high emissions. With appropriate control, it is also possible to react to power peaks with the help of a buffer storage tank.
At VWE, we use neural networks to predict the future heat demand of your district heating networks. The specifically adapted model uses historical data and other parameters (e.g. outside temperature) to calculate the expected power consumption. This prediction enables you to optimally use the buffer storage tank and reduces the operating time of the peak load boiler. In addition, your biomass boiler is operated more consistently and more quietly.
Use case measured value validation
The validation of measured values is essential to ensure the efficient operation of an automated system. Sensor failures and incorrect readings lead to suboptimal control and thus inefficiency. Artificial intelligence enables the validation of measured values without the use of additional hardware in the form of redundant sensors.
For example, a VWE regression model based on artificial intelligence is used to validate the measured value of an O₂ probe. The residual oxygen content measured with this probe is the most important source of information for combustion control. Accordingly, the correction of an incorrect measured value – for example due to a drifting sensor – is of great importance.
We also work with a neural network to calculate the boiler output, which is trained with purely flue gas-related input data (independent of the water side). This makes it possible to bridge brief failures of the heat meter or to validate the sensor output value.
Would you like to find out more? Should we call you or would you like to receive further information by e-mail?
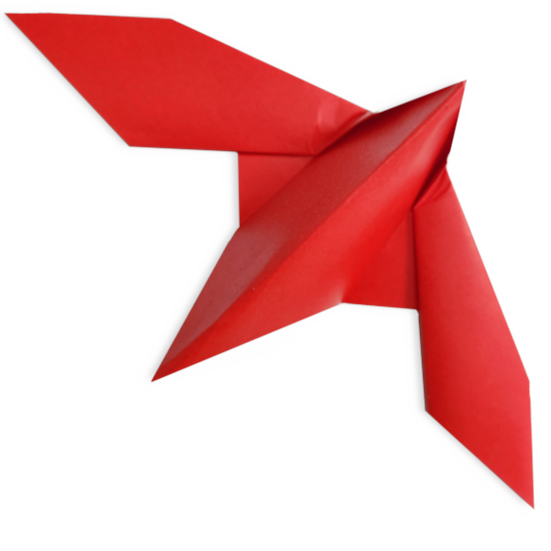
Further information:
